Bulletin – December 2018 Australian Economy Which Firms Get Credit? Evidence from Firm-level Data
- Download 481KB
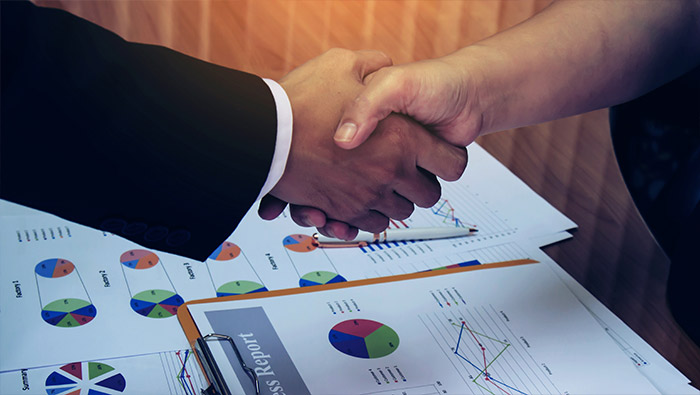
Abstract
To improve our understanding of how lenders assess firms' creditworthiness, this article relates the characteristics of firms to whether their applications for credit were approved. We find evidence to suggest that firms with relatively low profitability, high debt servicing burdens or limited credit histories were less likely to have their applications approved than other comparable firms. However, the decision to approve an application for credit also appears to be influenced by a range of other unmeasured factors, which possibly reflects the complexity of the approval process in practice.
Background
Firms need access to credit for a variety of reasons, including to finance: investment and innovation; their ongoing operations; and mergers and acquisitions. Although these activities can also be funded through other sources, such as equity raisings, bond issuance or retained earnings, credit from financial institutions accounts for a large share of firms' funding in Australia (Connolly and Jackman 2017). Moreover, credit from financial institutions is a particularly important source of funding for small or young firms. They are unlikely to be able to access capital markets, and may not have had time to accumulate sufficient earnings to fund these activities themselves. Therefore, changes in the availability of credit, such as through changes in the way financial institutions assess firms' credit applications, have the potential to affect economic growth.
From the perspective of lenders, and the financial system more broadly, lending to firms tends to be riskier than housing lending; loans to firms were the major source of credit losses for Australian banks during both the 1990s recession and the 2007/08 global financial crisis (Rodgers 2015). Similar patterns have been observed overseas, with business loans often accounting for the majority of bank loses during crises (Kragh-Sørensen and Solheim 2014). Changes in the way lenders assess applications for credit and, in particular, the degree of risk that they are willing to accept, can therefore also have important implications for financial stability. This can, in turn, also have flow-on effects on the economy. For example, lending standards that are overly lax may boost short-term economic growth, but, if they lead to a significant deterioration in asset quality and losses for banks, they may ultimately result in a contraction in credit supply and weaker economic growth over the medium term. That said, changes in the availability of credit can also affect the economy independently of their implications for financial stability, as overly tight lending standards could be harmful to the economy if they restrain investment and therefore productivity growth.
Who receives credit is therefore important not only to individual firms, but also to lenders and policymakers. In particular, changes in the availability of credit can pose risks to the financial health of lenders, the stability of the financial system and the economy. To improve our understanding of how lenders assess firms' applications for credit, we use firm-level data to examine what firm characteristics are most likely to be associated with applications being approved. We also assess whether these factors have changed over time.
Data
This article uses firm-level data from the Australian Bureau of Statistics (ABS) Business Longitudinal Analysis Data Environment (BLADE) database to analyse the determinants of firms' access to credit.[1] We use two types of data from the BLADE database. The first comes from the Business Characteristics Survey (BCS), while the second comes from firms' income tax returns.
The BCS contains information on whether firms applied for credit (debt finance) or for equity finance and, if they did apply, whether they received it and the reason for their application. The BCS covers around 10,000 listed and unlisted firms each financial year from 2005/06 to 2014/15. As a result, these data can to provide unique analytical insights based on a very comprehensive sample of firms, but they do not provide information on more recent developments.[2]
In any given year, around one in four firms in the BCS sample applied for credit, although the share has declined somewhat over the sample period (left panel Graph 1). This trend is consistent with the broad deleveraging of Australian firms since the financial crisis and the increasing tendency for firms to finance investment using internal funds (RBA, 2014; Fang, Kosev and Wakeling 2015; Connolly and Jackman 2017). Of those that did apply, on average around 90 per cent were successful in obtaining credit.[3] While this acceptance rate is high, it may in part reflect the fact that many firms that anticipate that they would not receive credit did not apply in the first place. In this sense, most of the analysis presented in this article should be interpreted as providing insights into what factors are most correlated with firms' access to credit, conditional on them applying. It is possible that this might not be representative of what factors are most important for lenders' decisions about whether to supply credit to firms in general. We attempt to address this concern in the final section of the article. The share of firms having their applications approved decreased around the time of the global financial crisis (right panel Graph 1). This could suggest that lending standards tightened, or that firms became riskier (or both).
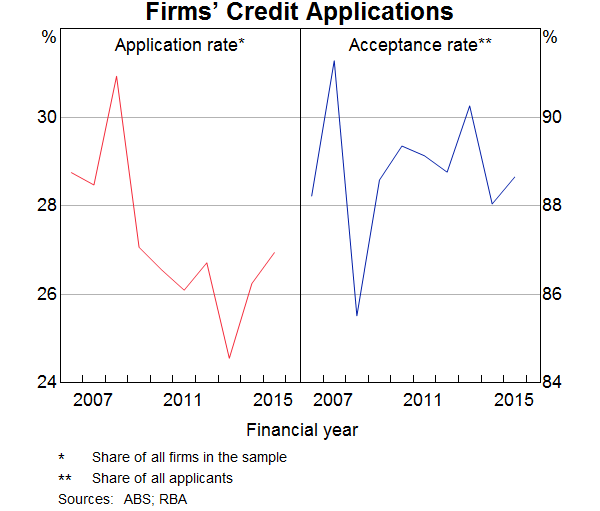
We match the BCS data to balance sheet data from firms' income tax returns.[4] The balance sheet data are used to construct a number of financial ratios that are commonly identified as being associated with a higher risk of default, and that we might therefore expect to be considered by lenders in assessing loan applications. These indicators are outlined below. These measures capture firms capacity to repay and to some extent the amount of capital they have put into the business, but will not capture some other factors that might affect the lenders' decisions, like the owner's ‘character’ and reputation, or the collateral they can provide (Connolly, La Cava and Read 2015).
Indicators of firm riskiness
In assessing a firm's application for credit, lenders are likely to place a high weight on the firms' capacity to repay. There is a large body of literature linking firms' capacity to repay loans and to avoid bankruptcy more generally, to the health of their balance sheet, which is often measured using financial ratios (see Altman (1983); Scott (1981); Ohlson (1980); Bunn and Redwood (2003); Vlieghe (2001); Bhattacharjee et al (2009); Kenney, La Cava and Rodgers (2016)). We consider a number of such ratios in our analysis:
- Gearing ratio (ratio of debt to equity) captures how much debt the firm has compared to its equity. Companies that have relatively high gearing are more vulnerable to asset devaluations as they have a relatively small equity buffer. This makes them more likely to go bankrupt and default. Moreover, in the event of default the lender is likely to experience a larger loss if the firm is highly geared because there will be fewer assets to liquidate. As such, all else being equal, firms with high gearing are riskier and may be less likely to receive additional credit. While gearing is usually measured as the ratio of debt to equity, we use debt to assets due to difficulties in measuring equity from the data. Also, debt is defined as liabilities less trade creditors, as debt is not reported separately in the data.
- Debt-servicing ratio (DSR; ratio of net interest expense to profits) captures how much of the firm's profits go towards meeting its debt obligations. If a firm has a high DSR it is more vulnerable to unexpected decreases in cash flows. All else being equal, firms with higher DSRs are riskier, and may be less likely to receive credit.
- Returns on assets (ROA; profits divided by assets) captures the firm's profitability. Firms with low ROA tend to have low cash flows. While a firm can survive without making a profit for some time, over the long term, survival is not possible without profit. Hence, a firm with a low ROA is more likely to cease operations due to poor performance and, in the process, potentially default on their debt. Given the increased default risk, firms with low ROAs may be less likely to receive credit.
- Liquidity ratio (ratio of cash to current liabilities) measures a company's ability to repay its current liabilities (obligations payable within the coming year) as they come due. All else being equal, a lower ratio implies that a firm will have more trouble meeting its financial obligations, including debt payments or trade creditors, and is therefore likely to be associated with having a higher default risk and lower chance of receiving credit. As firms do not report their cash holdings in their income tax returns, we proxy for cash as current assets less inventories and debtors.
In all cases, our measure of profits is income less all expenses except for interest expenses and income tax expenses.
Firms in different industries may have systematically different financial characteristics, such as indebtedness and profitability. Consequently, interpreting the riskiness of a firm by looking at the levels of these ratios, without comparing it to an industry benchmark, may be misleading. For instance, firms in some industries may have relatively high gearing ratios but may be able to sustain these through consistent cash flows (see, for example, Black et al (2012)). To account for this, we construct industry benchmarks using firm-level balance sheet data on all businesses in the BLADE database (not just those included in the BCS). For each firm we then focus on where its ratio sits relative to all firms in its industry.[5]
In addition to balance sheet variables, a number of other characteristics have also been shown to be associated with firm failure and default risk. For example, newer and smaller companies are often found to be more likely to fail, potentially reflecting trial and error among start-ups (e.g. Jovanovic 1982), or less consistent cash flows and customer bases. The legal or ownership structure has also been shown to be important (e.g. Kenney et al (2016)). This might reflect differences in the degree of imperfect information about the financial health of firms with different legal structures, whether between the owner and the manager of the firm, or between the firm and the lender.
The Relationship between Acceptance Rates and Firm Characteristics
We start by describing whether firms with certain characteristics were more or less likely to receive credit (conditional on having applied), and whether this has changed over time. To build on the insights from this descriptive analysis, we then use a regression framework to more formally estimate the relationship between these characteristics and the probability of obtaining credit.
Financial ratios
Firms with very high gearing ratios relative to others in their industry were less likely to receive credit than firms with moderate levels of gearing (Graph 2, upper panel). For example, of those firms with gearing ratios between the 40th and 60th percentiles of their industry's distribution, 96 per cent had their application approved, compared to 93 per cent of firms with gearing ratios above the 70th percentile of their industry's distribution. This is consistent with higher gearing being associated with higher risk. However, firms with very low gearing also appear to be less likely to obtain credit. One explanation may be that these firms were less likely to have pre-existing relationships with financial institutions or established credit histories, which may have made it more difficult for the lender to assess their creditworthiness. Past research has documented the importance of existing relationships in lending (e.g. Berger and Udell (2002); Banerjee, Gambacorta and Sette (2017) and Petersen and Rajan (1994)).
Firms with very low liquidity ratios relative to others in their industry also had a slightly lower probability of obtaining credit than firms with liquidity ratios around the middle of their industry's distribution (Graph 2, lower panel). This is consistent with firms with low liquidity being riskier. While the data also suggest that firms with very high liquidity ratios were less likely to have their applications accepted, the sample of very liquid firms is small which likely reflects the fact that very liquid firms may be less likely to apply for finance in the first place. Even if this finding holds true, it likely reflects some latent characteristic of these firms rather than their liquidity ratios per se. For example, firms with volatile and risky cash flows may be less likely to receive credit, and will also be likely to hold a large amount of liquid assets to buffer against unexpected liquidity shocks.
The data also show that firms with high profitability relative to others in their industry had a higher probability of obtaining credit than less profitable firms, while firms with relatively high DSRs were less likely to receive credit (Graph 3). This confirms that those that can more easily meet their debt obligations using internal cash flows were more likely to receive credit, as expected.
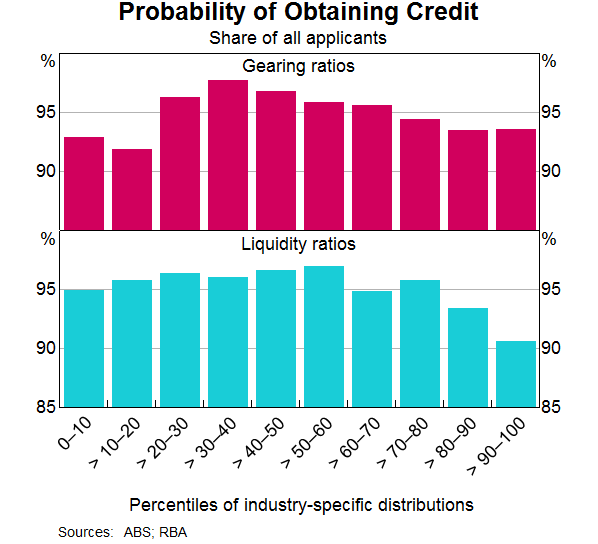
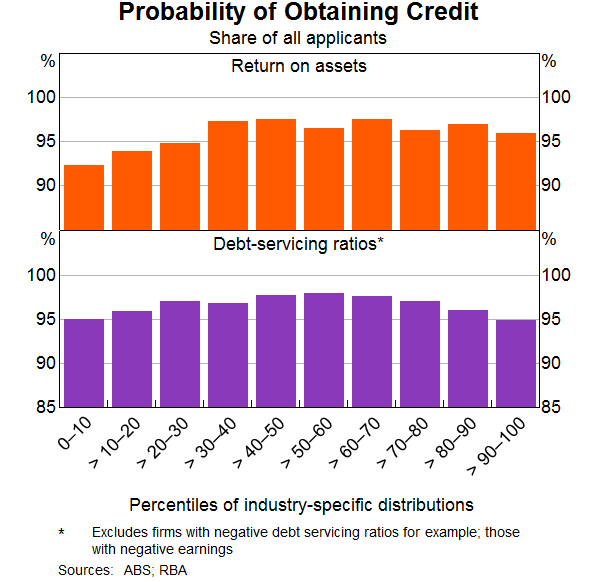
It is noteworthy that the relationship between the financial ratios and the probability of receiving credit does not appear to be linear. For example, firms with DSRs around the 30th percentile of their industry distributions appear to have been just as likely to receive credit as firms with DSRs around the 50th or 70th percentiles. However, a smaller proportion of firms with DSRs above the 80th percentile of their industry distributions received credit. This suggests that these ratios only become relevant to lenders when they move beyond a certain threshold. We carry this insight over to our regression analysis below.
Age and legal structure
Young firms, defined as firms that are five years old or younger, were less likely to have their applications for credit accepted (Graph 4).[6] Again, this is consistent with our expectations, as younger firms are generally found to be more likely to fail, and are less likely to have an extensive credit history that lenders can assess.
A firm's legal structure also seems to be correlated with the likelihood that its application for credit will be approved. In particular, incorporated firms were more likely to have their applications for credit accepted, compared to unincorporated firms. As noted above, this could reflect differences in the degree of imperfect information about the financial health of firms with different legal structures. Alternatively, the lower acceptance rates may instead reflect differences in the observable characteristics of incorporated and unincorporated firms, such as their size or age, rather than their legal structure per se. This is something we test – and find evidence for – in our regression framework.
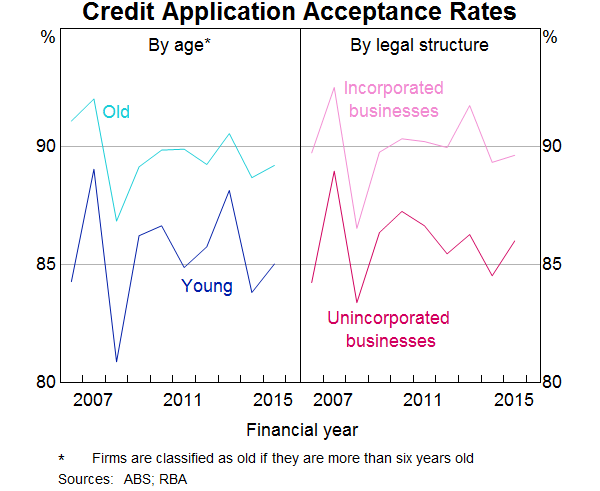
Regression Results
The preceding analysis provides an indication of some of the variables that might be important in predicting whether a firm receives credit (conditional on applying), and therefore what indicators lenders consider when assessing credit applications. But to identify the relative importance of each individual variable in this decision (after controlling for the influence of the other variables), we need to move to a regression framework. For example, a regression allows us to establish whether the lower approval rate for unincorporated firms is still evident after taking into account the fact that these firms also tend to be small.
Baseline regression
The baseline regression seeks to explain how the probability of each individual firm receiving credit varies based on a range of potential explanatory factors. These explanatory factors include those that vary across firms and some that are common to all firms but vary over time. The firm-specific explanatory factors are the financial ratios discussed above – namely, gearing, DSR, liquidity ratio and the ROA – as well as other firm characteristics, such as the age, size, industry and the legal structure of the firm. Finally, we also include survey information on the reason why the firm applied for credit. The explanatory factors that are common to all firms but vary over time are captured by including dummy variables for each year (year fixed effects).[7] Technical details on the model's specification and results are included in Appendix A.
As noted above, the relationship between the financial ratios and the probability of having an application for credit accepted does not appear to be linear. We therefore identify threshold percentiles for each ratio based on the earlier analysis, and incorporate information on whether the firm in question breached each threshold.[8] For example, a firm breaches the DSR threshold if its DSR is above the 80th percentile of its industry's distribution.[9] As the 80th percentile could be quite different in each industry, this allows for industry-specific benchmarks.
It should be noted that there is a range of potentially important firm-specific determinants of access to credit that cannot be included in the regression due to a lack of data. In particular, we have no information on the type of borrowing – for example, whether the firm is applying for a credit card or a term loan – or the size of the loan sought. We also do not know whether the firm is applying for a new loan or attempting to refinance an existing loan. And we have no information on whether the firm offered real estate as collateral, which can be particularly important for small and medium firms (Connolly and Bank 2018; Connolly et al 2015). The exclusion of these variables has two important consequences. First, the model is only able to explain a small share of the overall variation in the probability of an individual firm receiving a loan, meaning that the results are best interpreted as providing information on the relative importance of the measured variables rather than as an explanation of lending decisions as a whole. Second, to the extent that the excluded factors influence both the probability of getting credit and the financial ratios that are included as explanatory variables, caution should be applied in interpreting the results as implying a causal relationship. Some of these factors might also influence the likelihood of applying in the first place, which we consider in the next section.
Bearing these caveats in mind, we find that DSRs and ROAs are associated with the probability of having an application for credit accepted or rejected. In particular, the probability of receiving credit was one and a half percentage points lower for firms with high DSRs (above the 80th percentile of the industry-specific distribution) relative to comparable firms with lower DSRs. Similarly, firms with very low ROAs (below the 30th percentile of the industry-specific distribution) tended to have an acceptance rate that is around one and a half percentage points lower than comparable firms without low ROAs.
Firms with very low gearing ratios (below the 20th percentile) were three percentage points less likely to receive credit than those without very low gearing ratios. This suggests that having previous relationships with lenders, or at least some credit history, improves access to finance. This is consistent with Petersen and Rajan (1994), who find that having close ties with the lender increases the availability of credit to small firms. However, it is also possible that the result at least partly reflects omitted factors – such as a lack of real estate collateral – which might reduce both a firm's probability of obtaining credit and their gearing ratio. In contrast, having a high gearing ratio (above the 70th percentile) does not appear to be associated with decline in the probability of receiving credit, nor does having low liquidity ratio (below the 10th percentile), as neither has a statistically significant relationship with the probability of receiving finance.
Focusing on other characteristics of firms, we find that larger firms were more likely to receive credit, consistent with previous research that finds that large firms are less likely to fail (e.g. Kenney et al 2016). However, the estimated sensitivity is reasonably low. To put it into context, the 25th percentile of the asset distribution equates to around $5 million in assets while the 75th percentile equates to $100 million in assets. After controlling for the other included variables, a firm with $100 million in assets had a probability of having its loan approved just one percentage point higher than a comparable firm with $5 million in assets. Nevertheless, after controlling for size, young firms and unincorporated firms were no less likely to receive credit than comparable older firms and incorporated firms, respectively. This suggests that the earlier findings from Graph 4 were driven by the fact that young firms and unincorporated firms tend to be smaller.
Information on the purpose of the credit application suggests that firms that applied to fund physical capital investment were more likely to receive credit, while firms that applied to invest in IT systems were less likely to receive credit. For instance, firms that applied for credit to fund physical capital tended to have an acceptance rate that was one and a half percentage points higher than those that applied for other reasons, while firms that applied to invest in IT had an acceptance rate that was one half of a percentage point lower than firms that applied for other reasons. This is consistent with the fact that physical capital can be used as collateral and liquidated relatively easily in case of default, unlike IT and other intangible assets. It could also help to explain why firms have generally lowered their gearing and focused more on internal financing in recent years. As investment in intangibles and IT rises, firms may shift towards external equity funding, or internal funding, instead of debt funding, as it is a more suitable funding instrument (Cecchetti and Schoenholtz 2018). Unsurprisingly, firms that applied for credit in order to avoid failure were less likely to receive credit.
Finally, we examine the estimated year effects. These show how the probability of receiving credit has changed over time, on average across all firms, after controlling for firm-specific characteristics. In this sense they can be interpreted as an indicator of the availability of credit, or lending standards. However, there are two important caveats that are relevant to their interpretation. First, the year effects could also reflect aggregate trends that are not associated with lending standards. For example, we have no information on what type of credit firms are applying for. If firms were to shift from applying for credit cards to applying for term loans, and lenders were more likely to accept credit card applications, the average probability of acceptance for a given set of firm characteristics would decline. Such a trend would be impossible to distinguish from a tightening of lending standards in our model. Second, lending standards are multifaceted and changes in these standards are not likely to be reflected solely in changes in the probability of receiving credit. In particular, lenders may vary the price or non-price conditions associated with a given debt facility without necessarily altering the probability of approving it. For instance, a lender that has tightened its lending standards may be just as willing to lend to a firm with high gearing as previously, but may now require a higher interest rate to compensate them for the risk, or offer a shorter loan term. This latter caveat is likely to make it harder to find evidence of a change in lending standards, and so in this sense any evidence we do find could be considered to represent a lower bound.
The year fixed effects provide some tentative evidence that it has become harder for firms, on average, to obtain credit since the 2007/08 global financial crisis (Graph 5). This is consistent with a tightening of lending standards and decreased availability of credit in the post-crisis period. However, it should be noted the evidence is fairly weak, as most of the year effects are not statistically significantly different from zero.
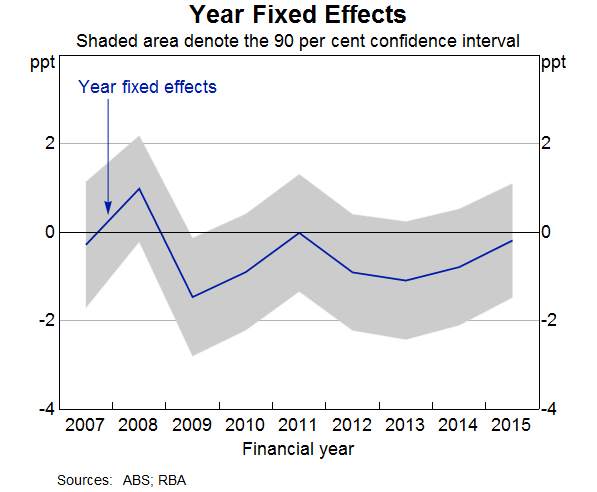
Another way that lending standards may have changed is that lenders could have begun to place a higher weight on certain risk metrics, such as the financial ratios. This would not necessarily show up clearly as a ‘general’ tightening of lending standards, as captured by the year-fixed effects. To test for this, we allowed the weights lenders place on the financial ratios to change from 2009/10 onwards. When doing so, we find statistically significant evidence that lenders have placed more weight on DSRs, liquidity and profitability in the post-crisis period. More precisely, we find that in the post-crisis period, breaching the thresholds for these ratios was associated with a larger decline in acceptance rates.
Accounting for sample selection
The analysis up to this point has focused only on firms that apply for credit. As such, it provides evidence on the factors that are associated with a firm receiving credit, conditional on them having first made the choice to apply for it. However, this might not necessarily provide a complete picture of what lenders care about when assessing loan applications, as lending standards are likely to influence firms' decision to apply for credit, as well as the likelihood that applications will be approved.
For example, assume that lenders are less likely to give credit to a firm with a high DSR, and that firms know this. In this case, firms with high DSRs may be less likely to apply for credit, and the subset of firms with high DSRs that do apply are more likely to be those that desperately need credit to survive and so are willing to apply even though they know their chances of success are low. These firms would have a particularly low probability of getting credit as, not only do they have high DSRs, but they are also about to fail. In such a scenario, the model presented above might overstate the importance of the DSR in explaining lenders' decisions to reject credit applications, as it will conflate these two sources of risk. This is an example of the so-called ‘selection bias’.
To try to ameliorate this problem, we estimate a sample selection model (see Appendix B for details and a table of results). The intuition behind these models is that by explicitly modelling selection (i.e. whether or not a firm applies for credit), we can capture unobservable factors like those discussed above, and account for them when estimating our model of application acceptance.
There is some evidence that the magnitudes of the estimated relationships in the baseline regression model are affected by sample selection issues. But the results from the sample selection model are nevertheless qualitatively very similar to those discussed above. Specifically, low gearing and profitability are still negatively related to a firm's chances of getting credit. Having a high DSR is still associated with a lower probability of receiving credit, but the size of the effect is now smaller.
In addition to providing a robustness check for the baseline model, the sample selection model also provides some insights into the factors that were associated with firms' decisions to apply for credit. Specifically, firms with high DSRs and high gearing appear to have been more likely to apply for credit. This might reflect the fact that these firms were more likely to need to apply to refinance existing facilities. Alternatively, it could also reflect the fact that these firms have some unobservable characteristics that makes them more likely to receive credit, such as having property to offer as collateral. This could lead to higher gearing, as they were more likely to receive credit in the past, and create a greater incentive to apply again, as they think they will receive credit. Meanwhile, larger firms were more likely to apply for credit, while older firms were less likely to apply for credit. The latter finding is consistent with other research, which has found that young firms tend to be more highly geared than older firms (Hambur, La Cava and Watson (forthcoming)).
Conclusion
Firms' access to credit is important for their ability to expand, while lending to firms accounts for a sizeable share of Australian banks' total lending. Credit losses associated with these loans can have significant effects on the financial system and the real economy. As such, it is important to understand how lenders assess firms' credit applications. We find that a firm's financial health, as measured by certain financial ratios, appears to be important in determining whether or not they will have their application for credit accepted. In particular, firms with relatively low profitability or high DSRs appear less likely to have their applications approved. There is also some evidence that firms with little credit history, as captured by a lower gearing ratio, are less likely to receive credit.
Appendix A: Baseline Regression
To assess which characteristics of firms are associated with firms having their applications for credit approved, we estimate a model of the following form:[10]
where is a dummy variable which denotes whether firm i obtained credit at time t. We do not include firm fixed effects as most firms only appear in the sample once. Year fixed effects are denoted by .
The remaining right-hand side variables can be split into two groups: (i) balance sheet variables, including the financial ratios (gearing, DSR, liquidity and ROAs), firm size (the log level of assets), and age; and (ii) dummy variables related either to firm characteristics or to the reasons for applying for credit, which we collect in the vector . Specifically, consists of:
- Industry: 1 if goods-related industry, 0 otherwise.[11]
- Company: 1 if firm is incorporated, 0 otherwise.
- Capital investment: 1 if reason for applying was to invest in physical capital, 0 otherwise.
- IT investment: 1 if reason for applying was to invest in IT, 0 otherwise.
- Cash flow: 1 if reason for applying for credit was to maintain liquidity, 0 otherwise.
- Survival: 1 if reason for applying is to ensure survival, 0 otherwise. Our baseline consists of firms that gave some other reason for applying for credit or those that did not list a reason for applying.
We lag all balance sheet variables (apart from age) by one year to ameliorate direct endogeneity issues.
For all financial ratios, we include an indicator variable which takes a value of 1 if a firm's ratio is above or below some threshold, and 0 otherwise. This was motivated by the graphical analysis, which suggested that the financial ratios only seem to affect probability of acceptance when they breach some threshold. The ratios used are outlined in Table A1, and were based on the graphical analysis. We also ran a specification which included the financial ratios in levels, and one where gearing entered as a piecewise linear function, similar to Gebauer S, R Setzer and A Westphal (2018). The results are broadly similar, though the threshold specification appears to provide better explanatory power.
Percentile (per cent) | |
---|---|
Low gearing | 20 |
High gearing | 70 |
ROA | 30 |
DSR | 80 |
Liquidity | 10 |
Sources: ABS; RBA |
Obtained credit | |
---|---|
Constant | 0.976*** (0.008) |
Low gearing | −0.032*** (0.009) |
High gearing | −0.006 (0.006) |
ROA | −0.014** (0.005) |
DSR | −0.012*** (0.004) |
Liquidity | 0.001 (0.006) |
Log(assets) | 0.004*** (0.001) |
Age | 0.000 (0.000) |
Good-related industry | −0.002 (0.004) |
Company type | −0.004 (0.005) |
Reasons for applying | |
Capital | 0.015*** (0.004) |
IT | −0.006 (0.004) |
Survival | −0.024*** (0.005) |
Cash flow | 0.001 (0.003) |
Other | 0.001 (0.004) |
Note: All balance sheet variables as well as application for equity
finance are lagged by one period. Our sample period is from financial
year 2005/06 to 2014/15 and we use yearly data. Heteroskedasticity-robust
standard errors are reported in brackets. ***, **, * denote statistical
significance at the 1, 5 and 10 per cent levels, respectively.
|
Appendix B: Sample Selection Model
We use a sample selection model in the vein of Heckman (1979). This involves creating a system of two equations: one for the variable of interest (acceptance or rejection), and an auxiliary equation for selection into the sample (apply or not). The results from the auxiliary equation are used to control for sample selection in the main equation, for example, by including the estimated probability of applying as an extra variable in the main regression.
The intuition behind these models is as follows. As discussed in the article, the sample selection problem comes about because those firms that enter the sample might tend to have some unobservable trait. By explicitly modelling selection, we can get some information on this trait, which we can then incorporate into our main regression to capture its effect on the probability of acceptance. To differentiate between the effect of the unobserved trait on the probability of acceptance, and the effect of the other variables on the probability of acceptance, we need to include additional instrumental variables in the auxiliary regression.[12] Ideally, they should help to determine whether or not a firm applies for credit, but not whether or not it receives credit, conditional on applying.
Our main equation is almost identical to the baseline regression discussed above. However, we exclude the ‘reason for applying’ variables, as these are not available for firms that do not apply for credit, and age, as it did not appear to significantly affect the probability of acceptance and as it represents a potential instrument. The auxiliary regression includes the same set of variables, but also includes two additional instrumental variables: age, and whether or not the firm applied for equity finance in the previous year. We concede that the assumption that these affect the probability of applying, but not of being accepted for credit is reasonably strong.
Table B1 contains the results from the auxiliary regression. Table B2 contains the results from the main regression, with and without the sample selection adjustment. The main and auxiliary equations were estimated jointly by maximising their joint likelihood function.
Applied for credit | |
---|---|
Constant | −0.669*** (0.030) |
Low gearing | −0.307*** (0.027) |
High gearing | 0.056** (0.022) |
ROA | −0.107*** (0.023) |
DSR | 0.112*** (0.021) |
Liquidity | 0.007 (0.026) |
Log(assets) | 0.105*** (0.004) |
Good-related industry | 0.110*** (0.015) |
Company type | 0.043** (0.019) |
Age | −0.018*** (0.001) |
Applied for equity finance | 0.706*** (0.024) |
Note: All balance sheet variables as well as application for equity finance are lagged by one period. Our sample period is from financial year 2005/06 to 2014/15 and we use yearly data. Heteroskedasticity-robust standard errors are reported in brackets. ***, **, * denote statistical significance at the 1, 5 and 10 per cent levels, respectively. |
Obtained credit | ||
---|---|---|
Corrected for Bias | Original Regression(a) | |
Constant | 0.951*** (0.010) |
0.973*** (0.009) |
Low gearing | −0.040*** (0.010) |
−0.034*** (0.009) |
High gearing | −0.002 (0.007) |
−0.004 (0.006) |
ROA | −0.017*** (0.006) |
−0.013** (0.006) |
DSR | −0.009* (0.005) |
−0.014*** (0.005) |
Liquidity | −0.008 (0.008) |
0.000 (0.007) |
Log(assets) | 0.005*** (0.001) |
0.004*** (0.001) |
Good-related industry | 0.002 (0.004) |
−0.002 (0.004) |
Company type | −0.003 (0.006) |
−0.004 (0.005) |
2008 | 0.015** (0.007) |
0.013* (0.007) |
2009 | 0.004 (0.008) |
−0.012 (0.008) |
2010 | −0.001 (0.008) |
−0.006 (0.008) |
2011 | 0.004 (0.010) |
0.003 (0.009) |
2012 | −0.004 (0.008) |
−0.006 (0.008) |
2013 | −0.004 (0.008) |
−0.008 (0.008) |
2014 | −0.008 (0.008) |
−0.005 (0.008) |
2015 | −0.001 (0.008) |
0.001 (0.008) |
(a) The time period has been restrained to post 2006 in order to do
a like-for-like comparison. |
Footnotes
Gabriela Araujo is from Financial Stability Department and Jonathan Hambur is from Economic Analysis Department. [*]
Includes loans (both from financial institutions and individuals), revolving credit facilities and trade credit. The results of these studies are based, in part, on ABR data supplied by the Registrar to the ABS under A New Tax System (Australian Business Number) Act 1999 and tax data supplied by the ATO to the ABS under the Taxation Administration Act 1953. Blade Disclaimer Notice [1]
The survey consists of a random sample of just under 7,000 firms, stratified by size and industry, as well as all firms employing over 300 people. For more details on the survey, and the data definitions, see <http://www.abs.gov.au/AUSSTATS/abs@.nsf/Lookup/8167.0Main+Features12016-17?OpenDocument>. [2]
We base our analysis on firms that had their application for credit either accepted or rejected. We do not include firms who reported that their applications for credit were still in progress. [3]
Balance sheet data are not available for sole traders, so they are excluded for much of the analysis. We also exclude firms in the finance industry and government-related entities. [4]
We also examined the probability of acceptance based on the levels of the ratios, rather than by comparing them to industry benchmarks. While the results were broadly similar when using unadjusted levels, benchmarking appeared to provide better explanatory power. [5]
There are data quality issues around the birth date of firms before 2000/01. Given that our time series begins in 2005/06, we treat all firms that are at least six years old as ‘old’. [6]
While the financial ratios are likely to be correlated, formal testing suggests that the estimated coefficients are unlikely to be heavily affected by multi-collinearity. [7]
We also ran a specification which included financial ratios in levels. The results are broadly similar, though the threshold specification appears to provide better predictive power. [8]
We could have identified different benchmarks for each industry (e.g. use the 70th percentile as the DSR threshold for firms in the agriculture industry, 90th percentile for the mining industry and so on). But for simplicity we apply a single percentile as the threshold. [9]
We present the results from this linear probability model as they are easier to interpret. The results from a logistic regression are similar in terms of both the sign and statistical significance of the coefficient estimates. [10]
Goods-related industries include: agriculture; mining; manufacturing; electricity, gas and water supply; construction; wholesale trade; retail trade; and transport and storage. Service-related industries include all other ANZSIC06 divisions except for financial services and public administration, which are excluded from the analysis. [11]
Otherwise the probability of applying will be co-linear with the other variables in the main equation, as it is a function of the exact same variables. [12]
References
Altman E (1983), ‘Financial ratios, discriminant analysis and the prediction of corporate bankruptcy’, The Journal of Finance, 23(4), pp 589–609.
Banerjee R, L Gambacorta and E Sette (2017), ‘The real effects of relationship lending’, BIS Working Papers, No 662.
Beaver W (1966), ‘Financial Ratios as Predictors of Failure’, Journal of Accounting Research, 4, pp 71–111.
Berger A and G Udell (2002), ‘Small Business Credit Availability and Relationship Lending: The Importance of Bank Organisational Structure’, Economic Journal, Royal Economic Society, 112(477), pp 32–53.
Bhattacharjee A, C Higson, S Holly and P Kattuman (2009), ‘Macroeconomic Instability and Business Exit: Determinants of Failures and Acquisitions of UK Firms’, Economica, 76, pp 108–131.
Black S, A Fitzpatrick, R Guttmann and S Nicholls (2012), ‘The Financial Characteristics of Small Businesses’, RBA Small Business Finance Roundtable, pp 25–32.
Bunn P and V Redwood (2003), ‘Company accounts based modelling of business failures and the implications for financial stability’, Bank of England Working Paper No 210.
Cecchetti S and K Schoenholtz (2018), ‘Financing intangible capital’, CEPR Policy Portal.
Connolly E and J Bank (2018), ‘Access to Small Business Finance’, RBA Bulletin, September, pp 1–14.
Connolly E and B Jackman (2017), ‘The Availability of Business Finance’, RBA Bulletin, December, pp 55–66.
Connolly E, G La Cava and M Read (2015), ‘Housing Prices and Entrepreneurship: Evidence for Housing Collateral Channel in Australia’, RBA Small Business Conditions and Finance, pp 115–148.
Fang A, M Kosev and D Wakeling (2015), ‘Trends in Australian Corporate Financing’, RBA Bulletin, December, pp 29–38.
Gebauer S, R Setzer and A Westphal (2018), ‘Corporate debt and investment: A firm-level analysis for stressed euro area countries’, Journal of International Money and Finance, 86, pp 112–130.
Heckman J (1979), ‘Sample Selection Bias as a Specification Error’, Econometrica, 47(1), pp 153–161.
Jovanovic B (1982), ‘Selection and the Evolution of Industry’, Econometrica, 50(3), pp 649–670.
Kragh-Sørensen K and H Solheim (2014), ‘What do banks lose money on during crises?’, Norges Bank Staff Memo, Financial Stability, No 3.
Kenney R, G La Cava and D Rodgers (2016), ‘Why Do Companies Fail?’, RBA Research Discussion Paper No 2016-09.
Ohlson J (1980), ‘Financial Ratios and the Probabilistic Prediction of Bankruptcy’, Journal of Accounting Research, 18(1), pp 109–131.
Petersen M and R Rajan (1994), ‘The Benefits of Lending Relationships: Evidence from Small Business Data’, Journal of Finance, 49(1), pp 3–37.
RBA (2014), ‘Financial System Inquiry’, Submission to the Financial System Inquiry, Reserve Bank of Australia, March.
Rodgers (2015), ‘Credit Losses at Australian Banks: 1980–2013’, RBA Research Discussion Paper No 2015-06.
Scott W (1981), ‘Organizations: Rational, Natural and Open Systems’, Englewood Cliffs, New Jersey: Prentice-Hall.
Vlieghe G (2001), ‘Indicators of Fragility in the UK Corporate Sector’, Bank of England Working Paper No 146.